---
### Download continuous seismic data 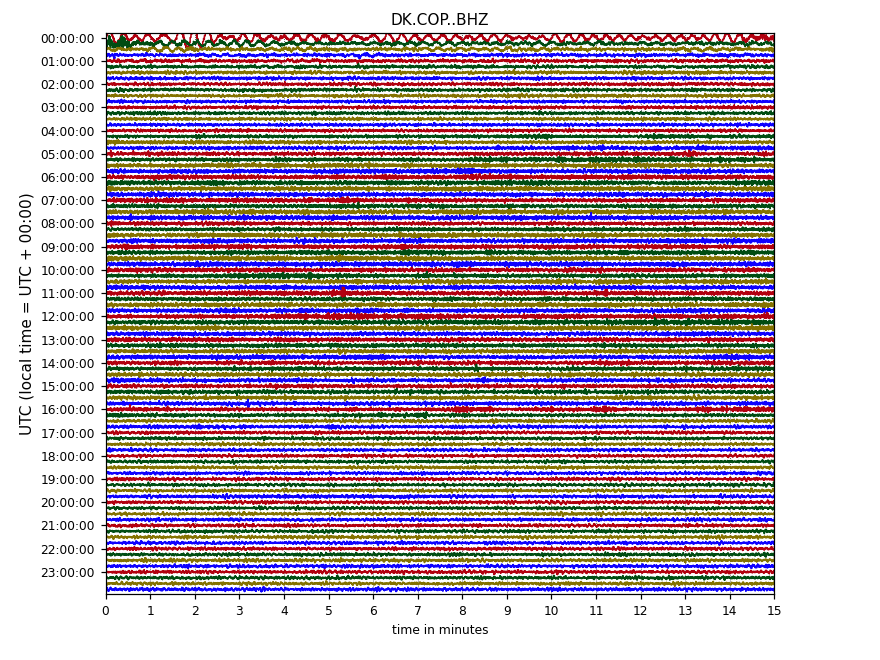
